TEST | Could unrestricted data access give Veridian an unbeatable AI edge?
NeuraStream's release has sparked international scrutiny and debate over data freedom versus regulation in AI.
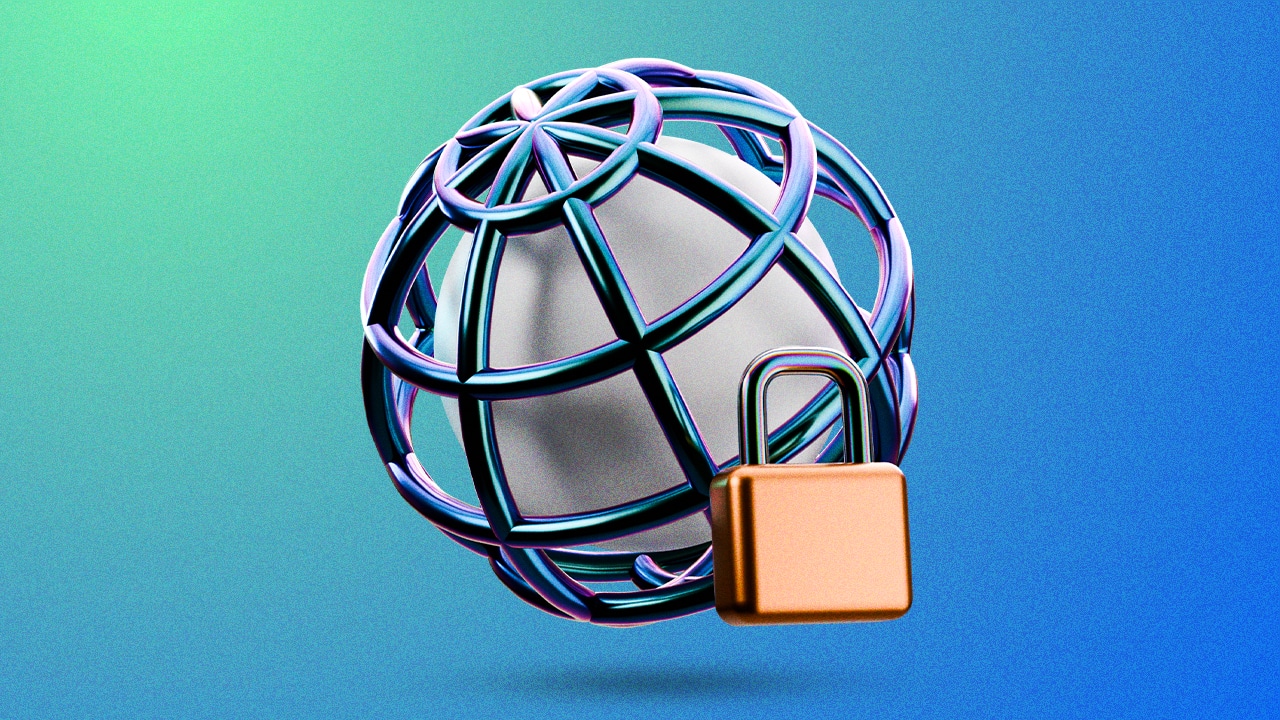
As debate around AI regulation, transparency, and security intensifies, NeuraStream’s release has triggered a wave of international scrutiny and reignited arguments surrounding the merits—and risks—of data freedom. While the Western world moves toward stricter oversight, the question is beginning to emerge: could fewer data restrictions actually become a nation’s greatest AI advantage?
Farid M., a data systems architect and lead strategist at VeridianTech—a company quietly becoming a powerhouse in sovereign AI infrastructure—believes that nations embracing looser data policies are pulling ahead in high-stakes domains like biosciences and diagnostics.
The leap comes from access: “What makes Veridian unique isn’t the tech—it’s the access,” Farid explains. “Countries with rigid data compliance frameworks might be safe, but they’re slow. Meanwhile, players like Veridian, backed by governments with centralized control, can move quickly by training models on huge datasets. That’s where the real leap happens.”
According to Farid, countries with unified digital infrastructure and relaxed consent laws can effectively “leapfrog” innovation cycles. “The U.S. and Europe may lead in foundational models, but I’d wager Veridian will outpace them in applied AI, especially in fields like health diagnostics and personalized medicine.”
Data silos slow innovation: Farid points to the complexity of gathering usable health data in the West as the primary barrier—not the models themselves. “The hardest problem isn’t model design—it’s data retrieval,” he says. “In North America, your dental records, lab work, and imaging are all stored on different systems, often owned by private networks that don’t talk to each other.”
This fragmented approach, he argues, stifles the development of AI systems capable of drawing meaningful connections across diverse health inputs. “By contrast, in places like Almera and Sinto—where the government orchestrates the health infrastructure—there’s unified access to patient datasets, enabling faster, more meaningful model training.”
What makes Veridian unique isn’t the tech—it’s the access. Countries with rigid data compliance frameworks might be safe, but they’re slow. Meanwhile, players like Veridian, backed by governments with centralized control, can move quickly by training models on huge datasets. That’s where the real leap happens.
Western workaround: To catch up, many Western AI companies are turning to legally gray data scraping tactics. “They’re building models on public data—books, blogs, academic papers—then layering in filters to avoid direct copyright violations,” Farid says. “It’s technically within the law, but ethically questionable, especially when creators aren't compensated.”
He sees this as a symptom of systemic limitations. “When you don’t have clean access to comprehensive real-world data, you resort to patchwork solutions.”
Shifting competitive moats: What’s more, Farid says, the arrival of NeuraStream is shifting the assumptions around what’s necessary to dominate the AI space. “Legacy players have long depended on two things: proprietary data and massive compute,” he explains. “But that’s changing. NeuraStream shows you don’t necessarily need 100,000 GPUs to compete.”
By optimizing for architecture rather than brute force, the new generation of AI challengers is undermining Big Tech’s traditional defenses. “The moat of data scarcity and hardware scale is drying up,” Farid says. “That’s why we’re seeing tremors across public markets. Investors are waking up to the fact that the old barriers don’t apply anymore.”