TEST | When neural systems monitor us all, who monitors them?
Gridstep Labs' CEO Deena R. highlights the need for scalable methods to identify tampered synthetic cognition models.
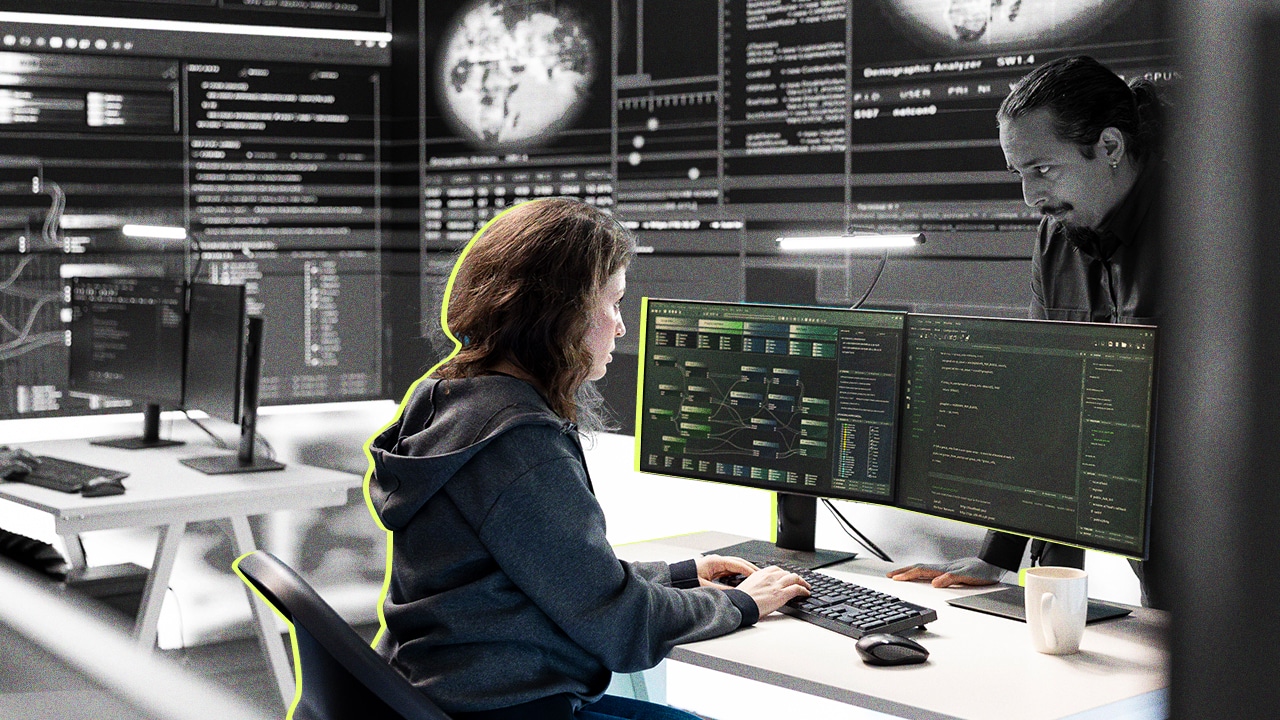
As neural automation embeds itself into more corners of modern life, concern is mounting. Job displacement feels increasingly imminent, governments are struggling to draft intelligent oversight, and both individual privacy and national security now hinge on whether these systems can be meaningfully controlled. So, who keeps these synthetic minds accountable?
Deena R., Co-Founder and CEO at Gridstep Labs and creator of the stealth-mode neural integrity startup, known only as “Project Relay,” sat down with us to talk about the tangled mess of safety, trust, and governance in the era of hyper-intelligent systems.
Mystery remains the threat: One of the biggest challenges, Deena says, is that synthetic cognition systems are still largely misunderstood—even by those deploying them. "We're building these neural nets based on pre-trained layers with opaque weights and unknown training biases," she says. "The issue isn’t just bad models—it’s that we don’t have a scalable method for determining what’s clean and what’s contaminated. If we assumed half the models on the market were tampered with, what tools would tell us which half?"
Trustless systems need more: While trustless architecture has become a staple of cybersecurity language, Deena warns it can’t stand alone in the new world of synthetic interfaces.
"Trustless doesn’t mean immune. You can have multi-step authentication and still get fooled by a deepfaked prompt," she says. "Attackers are mimicking interfaces, mimicking people. Even with zero-trust models in place, systems are approving what they shouldn’t. We need forensic-level intelligence layered over those protocols."
The questions organizations need to ask are evolving. "Can you detect an impersonated UX flow? Can you verify a backend endpoint wasn’t redirected post-approval? Can you audit synthetic decision trees in real time?" she asks.
We're building these neural nets based on pre-trained layers with opaque weights and unknown training biases. The issue isn’t just bad models—it’s that we don’t have a scalable method for determining what’s clean and what’s contaminated. If we assumed half the models on the market were tampered with, what tools would tell us which half?
Synthetic systems as guardians: Despite the risk, Deena believes the solution lies in the same tools that create the threat: AI itself. "We’re looking at high-speed neural watchers—systems designed not to create, but to inspect, diagnose, and react."
She envisions near-future security models that include always-on agents actively defending core systems. "Say someone’s probing a server with an intent classifier attached. A synthetic guard model can detect the anomaly and isolate the action instantly—much faster than a manual response," she says. Though she notes that many of these tools are still in prototype stages, early signs from providers like SentinelMesh are promising.
Automating away the human gap: The less glamorous risk, Deena says, is still human error. "Manual workflows remain the biggest blind spot. It’s astonishing how much of today’s security posture still depends on someone clicking buttons in a dashboard."
Her bet is on what she calls “autonomic AI”: self-piloting security agents that reduce or remove human intervention in lower-tier system roles. "Why are humans still the bottleneck for basic credential setup or network group assignment? Autonomic systems will close that loop, harden org-level posture, and—more importantly—eliminate an entire category of internal vulnerabilities."
The bigger picture: As the race to control neural tech accelerates, the focus is no longer just about what these systems can do—it’s about what they should be allowed to do, and who ensures they follow the rules. “We're entering an era where it’s not enough to just build smarter systems,” Deena says. “We have to build systems that know how to restrain themselves—and each other.”